Master CSMI: Proposition de maquette pour 2024-2028
- 1. Introduction
- 2. Socio-Economical and Research Context
- 3. Master CSMI: Current Track 2016-2023
- 4. Evolution of the Master CSMI
- 4.1. A change of name
- 4.2. English courses versus Courses in English
- 4.3. Introduction to Scientific Maching Learning
- 4.4. Blurring the line between Model driven versus data driven modeling and data assimilation
- 4.5. High Performance Computing
- 4.6. Courses
- 4.7. Skills set to be acquired by CSMI Students
- 4.8. Courses and Skills
- 5. ChangeLog
- Appendix A: Discussion on Networks and Pre and Post-Processing in Scientific Computing Courses
This document is also available in > PDF format. |
1. Introduction
We discuss here the evolution of the Master Track. It’s important to ensure that the program is meeting the needs of students and providing them with the skills and knowledge necessary for success in their chosen careers.
The main purpose of the CSMI Master’s program is to provide students with advanced skills in both mathematics and computer science, with a focus on modeling, simulation, optimization, signal and image processing, data mining, and high-performance computing. The program aims to equip students with the theoretical and practical knowledge necessary to tackle complex problems in the socio-economic world, and to prepare them for careers in research and development departments of companies, service companies, specialized consulting firms, or engineering positions in universities and public or private research organizations.
In real life work, the skills developed in the CSMI program are highly valued by employers in a wide range of fields. For example:
-
Modeling, simulation, and optimization skills are highly relevant in industries such as finance, energy, transportation, and healthcare, where companies must optimize complex systems to improve efficiency and reduce costs.
-
Signal and image processing skills are valuable in fields such as telecommunications, defense, and medical imaging, where companies must analyze and interpret large volumes of data.
-
Data mining skills are highly sought after in industries such as marketing, e-commerce, and finance, where companies must analyze large datasets to identify trends and patterns.
-
High-performance computing skills are valuable in many scientific and engineering fields, where companies must simulate complex systems or process large amounts of data in a timely manner.
Overall, the CSMI Master’s program is designed to prepare students for highly rewarding careers in a wide range of fields, where they can apply their skills to tackle complex real-world problems and make a positive impact on society.
We start by recalling the socio-economical impact study of mathemacs (EISEM in french) then a short presentation of the current Master track, then we discuss the evolution of the program, and finally we propose a new maquette for the program.
2. Socio-Economical and Research Context
2.1. National Context : EISEM report
The EISEM report was commissioned by the Agency for mathematics in interaction with entreprises and mathematical societies(AMIES) in 2015 and updated in 2022 by CNRS INSMI to evaluate the socio-economic impact of mathematics in France. The report highlights the crucial role that mathematics plays in driving innovation and competitiveness in a wide range of industries, and identified key technologies that are central to the needs of the socio-economic world.
The CSMI Master’s program at the University of Strasbourg is specifically designed to address these needs, by providing students with advanced skills in the key technologies identified by the EISEM report. For example, the program includes courses in modeling, simulation, optimization, signal and image processing, data mining, and high-performance computing, which are all key areas highlighted in the EISEM report.
By equipping students with these advanced skills, the CSMI program aims to prepare them for careers in research and development departments of companies, service companies, specialized consulting firms, or engineering positions in universities and public or private research organizations. These are all areas where mathematics plays a crucial role in driving innovation and competitiveness, and where the skills developed in the CSMI program can make a significant contribution.
The november 2022 version is available here.
Here are some key points of the latest report:
-
In 2019, 3.3 million salaried jobs, or 13% of all salaried jobs, were impacted by mathematics, contributing 381 billion euros in added value.
-
From 2012 to 2019, the share of salaried jobs impacted by mathematics in total salaried employment in France increased by almost 14%. Moreover, the contribution of mathematics to the country’s gross domestic product (GDP) grew by two percentage points, from 16% to 18%. *Almost half of the salaried jobs impacted by mathematics are concentrated in Ile-de-France (35%) and Auvergne-Rhône-Alpes (12%).
-
Sectors that heavily demand mathematics include: computer services (78% of jobs impacted by mathematics), scientific research and development (72%), production and distribution of electricity and gas (59%), telecommunications (59%), and manufacturing of computer, electronic, and optical products (57%). From 2016 to 2019, the share of jobs impacted by mathematics significantly increased in telecommunications (47% in 2016 vs. 59% in 2019), the pharmaceutical industry (41% to 44%), and financial and insurance activities (29% to 32%).
-
Five sectors account for half of the jobs impacted by mathematics: legal, accounting, management, architecture, engineering, control, and technical analysis services (15%); computer services (11%); financial and insurance activities (8%); commerce (8%); construction (6%).
-
There is a risk of a shortage of people with mathematical training, which companies fear could impact the country’s sovereignty. Economic actors are actively seeking three types of intermediate and advanced skills that are in short supply: advanced skills in mathematics development (often destined for R&D positions) and advanced skills in multidisciplinary use of mathematics (usually destined for R&D positions or data scientist roles).
The text highlights several areas where collaborations between the academic world of mathematics and businesses should be encouraged:
- Identification of Mathematical Skills
-
Businesses often don’t identify the mathematical skills that can be mobilized in the economic world. Therefore, efforts should be made to improve the understanding of the skills of PhDs in mathematics and how these can be applied in a business context.
- Increasing Visibility and Access
-
The visibility of and access to the scientific mathematical community are crucial factors for collaboration. Actions by the Agency for Mathematics in Interaction with Enterprises and Society (AMIES) that provide an interface between businesses and research laboratories, such as funding for exploratory first support (PEPS) projects and weeks of mathematical business studies (SEME), should be amplified and made known to all businesses.
- Enhancing Innovation
-
France is a nation of mathematics whose quality of training and research is regularly acclaimed, notably by the highest international awards (for example, the Fields Medal awarded to Hugo Duminil-Copin). About 33% of mathematics PhD graduates in 2017 specialized in fields immediately useful to businesses (numerical analysis and scientific computing, statistics, probabilities, and stochastic models). All mathematics PhD students acquire skills necessary for businesses, such as the ability to work on a complex, time-limited project by dividing it into projects with intermediate objectives, and the ability to communicate results.
2.2. Local and Regional Context: Cemosis
CSMI benefits greatly from its association with the local platform Cemosis at the University of Strasbourg. Cemosis plays a crucial role in fostering collaborations between mathematics, businesses, and other disciplines, creating a dynamic environment for innovation and knowledge exchange.
As a part of the regional European Digital Innovation Hubs (EDIH) network starting in Fall 2023, Cemosis will be at the forefront of accelerating the digital transformation in the Grand Est region. This network, led by Grand-Enov, aims to promote and support digital innovation across various sectors and industries. By being connected to this network, CSMI gains access to a wider ecosystem of expertise, resources, and opportunities.
The inclusion of Cemosis in the EDIH network reflects the recognition of its significant contribution to the digital landscape and its potential to drive innovation in the region. CSMI students can leverage this ecosystem to collaborate with businesses, engage in cutting-edge projects, and stay up-to-date with the latest technological advancements.
Overall, Cemosis and its involvement in the EDIH network further enhance the local and regional context of CSMI, positioning it as a program that not only prepares students with advanced mathematical and computational skills but also connects them with real-world opportunities and collaborations in the digital domain.
2.3. A Strong Local Research Ecosystem
Starting Fall 2023, the applied mathematics team and Cemosis at UNISTRA will actively participate in two significant initiatives: PEPR Numpex and PEPR AI. These collaborations bring several benefits to CSMI students, enhancing their learning experience and future prospects.
-
PEPR Numpex: As part of the PEPR Numpex initiative, the applied mathematics team and Cemosis will contribute to the methods, algorithms and the development of the software stack for exascale computing. This involves working alongside esteemed partners : CEA, INRIA, Ecole Polytechnique, and Sorbonne Université. The exposure of CSMI students to this project offers them the following benefits:
-
Cutting-edge Research: Students will engage in cutting-edge research and development efforts, gaining exposure to the latest advancements in exascale computing. This hands-on experience will deepen their understanding of advanced methods and algorithms in this field.
-
Collaboration Opportunities: Working with renowned institutions and experts in the field provides students with valuable opportunities for collaboration. They will be able to exchange ideas, network, and build relationships that can enhance their future career prospects.
-
Practical Applications: Participating in PEPR Numpex allows students to apply their mathematical and computational skills to address real-world challenges in exascale computing. This practical experience will equip them with a deeper understanding of the field and enhance their problem-solving abilities.
-
-
PEPR AI: The involvement of the applied mathematics team and Cemosis in focus projects on Partial Differential Equations (PDE) and Artificial Intelligence (AI) as part of the PEPR AI initiative brings additional benefits to CSMI students:
-
Interdisciplinary Learning: Students will have the opportunity to work at the intersection of mathematics, AI, and PDEs, fostering interdisciplinary learning. This exposure to diverse fields enhances their ability to tackle complex problems and widens their career prospects.
-
Research Opportunities: The collaboration with the Modeling and Control Team at the IRMA Laboratory opens avenues for research in mathematical modeling and control. Students can actively contribute to cutting-edge projects and make significant contributions to the field.
-
Skill Development: Engaging in PEPR AI projects allows students to develop advanced skills in PDEs, AI, and their integration. This unique combination of expertise positions CSMI students for high-demand roles in fields such as data science, machine learning, and computational sciences.
-
By participating in these initiatives, CSMI students gain exposure to state-of-the-art research, collaboration opportunities with renowned institutions, and practical applications of mathematics and computational sciences. These experiences enrich their learning journey, broaden their horizons, and prepare them for successful careers in emerging and impactful domains.
3. Master CSMI: Current Track 2016-2023
3.1. Description
The CSMI Master’s program is at the heart of the digital revolution, focusing on models, data, and algorithms. It aims to train students to be key players in the digital revolution, equipping them with cross-disciplinary skills in mathematics and computer science and a strong grasp of various application domains such as health, environment, economy, and micro-technology.
The program is designed to prepare students for the rapid technological changes and challenges in the digital world by providing them with the knowledge and skills needed in the areas of image processing, modeling, simulation, optimization, and high performance computing.
3.2. Main Components
3.2.1. Data and Machine Learning
This component covers the fundamentals of data analysis and machine learning. Students will learn about statistical methods, data analysis techniques, and machine learning algorithms. They will gain the ability to analyze and interpret complex datasets, and develop algorithms to learn from and make predictions or decisions based on data.
3.2.2. Modeling Simulation Optimisation
Modeling, Simulation, and Optimisation (MSO) is considered the third pillar of scientific progress and innovation, alongside experimentation and theory. In this component, students will learn about mathematical modeling, simulation techniques, and optimization methods. They will gain the ability to develop precise methods for MSO, which is increasingly important in the context of the growing importance of high-performance computing and Big Data technologies.
3.2.3. High Performance Computing
High Performance Computing (HPC) involves the use of supercomputers and parallel processing techniques for solving complex computational problems. In this component, students will learn about the architecture of high-performance computers, parallel programming techniques, and the design and optimization of high-performance algorithms.
3.2.4. Signal and Image Processing
Signal and image processing involves the analysis, interpretation, and manipulation of signals and images. In this component, students will learn about various methods and techniques for signal and image processing, including filtering, pattern recognition, and image enhancement. They will gain the ability to develop algorithms for processing and analyzing signals and images.
3.3. Course Summary and List
This table provides an overview of the lecture hours for each course in the first semester of the CSMI Master’s program.
Course | ECTS | CM | CI | TD | TP | TE |
---|---|---|---|---|---|---|
Algorithmique |
3 |
- |
28h |
- |
- |
- |
Base de données |
3 |
- |
28h |
- |
- |
- |
Analyse fonctionnelle |
3 |
- |
28h |
- |
- |
- |
C++ |
3 |
- |
28h |
- |
- |
- |
Calcul parallèle |
3 |
- |
28h |
- |
- |
- |
Calcul scientifique 1 |
3 |
- |
28h |
- |
- |
- |
Graphe 1 |
3 |
- |
28h |
- |
- |
- |
Modèles aléatoires |
3 |
- |
28h |
- |
- |
- |
Calcul scientifique 2 |
3 |
- |
28h |
- |
- |
- |
Langue |
3 |
- |
- |
16h |
- |
60h |
Anglais - S1 Master |
- |
- |
- |
16h |
- |
60h |
This table provides an overview of the lecture hours for each course in the second semester of the CSMI Master’s program.
Course | ECTS | CM | CI | TD | TP | TE |
---|---|---|---|---|---|---|
Traitement du signal 1 |
3 |
- |
28h |
- |
- |
- |
Projet |
3 |
- |
28h |
- |
- |
- |
Méthodes numériques EDP |
6 |
- |
56h |
- |
- |
- |
Optimisation |
6 |
- |
56h |
- |
- |
- |
Système d’exploitation |
3 |
- |
28h |
- |
- |
- |
Traitement et fouille de données |
3 |
- |
28h |
- |
- |
- |
Stage ou mémoire |
6 |
- |
- |
- |
- |
- |
This table provides an overview of the lecture hours for each course in the third semester of the CSMI Master’s program.
Course | ECTS | CM | CI | TD | TP | TE |
---|---|---|---|---|---|---|
Traitement du signal 2 |
3 |
- |
28h |
- |
- |
- |
Contrôle optimal |
6 |
- |
56h |
- |
- |
- |
Calcul scientifique 3 |
3 |
- |
28h |
- |
- |
- |
Méthodes numérique pour les EDP |
3 |
- |
28h |
- |
- |
- |
Compilation |
3 |
- |
28h |
- |
- |
- |
Projet |
3 |
- |
28h |
- |
- |
- |
Réseaux |
3 |
- |
28h |
- |
- |
- |
Incertitudes |
3 |
- |
28h |
- |
- |
- |
Graphe 2 |
3 |
- |
- |
- |
- |
- |
This table provides an overview of the lecture hours for each course in the fourth semester of the CSMI Master’s program.
Course | ECTS | CM | CI | TD | TP | TE |
---|---|---|---|---|---|---|
Stage |
27 |
- |
- |
- |
- |
- |
Langue S4 |
3 |
- |
- |
16h |
- |
60h |
Anglais - S3 Master |
- |
- |
- |
16h |
- |
60h |
The english courses are taken during the third semester. |
4. Evolution of the Master CSMI
There are several reasons why updating the Master track CSMI is beneficial. Here are a few reasons:
- Keeping up with changing industry demands
-
The world of mathematics and computer science is constantly evolving, and so are the needs of employers. By updating the Master track CSMI, we can ensure that our graduates have the skills and knowledge that are most in demand in the current job market.
- Attracting more students
-
Students are often drawn to Master’s programs that offer cutting-edge courses and relevant, practical training. By updating the CSMI program with new courses on high-performance computing, machine learning, and data assimilation, we may be able to attract more students who are interested in these fields.
- Strengthening industry partnerships
-
By incorporating projects with companies and internships throughout the program, as well as courses that are directly relevant to industry needs, we can build stronger partnerships with companies and potentially create more job opportunities for our graduates.
- Enhancing the reputation of the program
-
By offering a program that is up-to-date and relevant, we can enhance the reputation of the CSMI program and increase its visibility both nationally and internationally. This can help attract the best students and faculty to the program, and create more opportunities for collaboration and funding.
We aim at updating the Master track CSMI in several ways:
-
Updating the course offerings to reflect new developments in mathematics and computer science
-
Introducing new courses or modifying existing courses to better meet the needs of students
-
Increasing the number of courses taught in English to better prepare students for international settings
-
Providing more opportunities for practical experience, such as internships or industry partnerships
-
Incorporating more interdisciplinary coursework to help students understand the connections between mathematics and computer science and other fields
In particular, we propose to add courses on high-performance computing, machine learning, as these are all areas where there is high demand for skilled professionals. We also propose to introduce more interdisciplinary coursework, such as courses on scientific machine learning and data assimilation, to help students understand the connections between mathematics and computer science and other fields.
4.1. A change of name
The name of the Master track CSMI has been used for a few years now. Changing name is not a trivial matter. We can keep the name but change its meaning. It currently means : "Calcul Scientifique et Mathématiques de l’Information".
The term "Mathematics of Information" refers to a field of study that focuses on the mathematical principles, theories, and methods used in the analysis, processing, and communication of information. It involves the application of mathematical concepts and techniques to various aspects of information processing, including data compression, coding theory, information theory, cryptography, error correction, signal processing, and more. The master track CSMI is not focused on these topics anymore and we should be careful that the name does not mislead students.
We can make the following changes:
- Calcul Scientifique et Mathématiques de l’Innovation
-
This translates to "Scientific Computing and Mathematics of Innovation" in English. It emphasizes the focus on leveraging mathematical and computational techniques to drive innovation in various fields.
- Calcul Scientifique et Mathématiques de l’Industrie
-
This translates to "Scientific Computing and Mathematics of Industry" in English. It highlights the application of mathematical and computational methods in industrial settings, addressing challenges and optimizing processes in various industrial sectors.
- Calcul Scientifique et Mathématiques de l’Ingénieur
-
This translates to "Scientific Computing and Mathematics of Engineering" in English. It emphasizes the application of mathematical and computational techniques in engineering disciplines, supporting the development of innovative solutions and technologies.
As the person responsible for the Master CSMI my preference goes to the first proposition. |
4.2. English courses versus Courses in English
In response to student feedback, the Master CSMI program has implemented changes to the curriculum regarding English language courses. The previous offering of two 3 ECTS English courses in Semester 1 and Semester 3, aimed at enhancing English skills, has been eliminated due to poor reception among students.
Instead, the program now includes at least 2 or 3 standard courses per semester taught in English, along with projects that require students to write reports and deliver presentations in English. This modification aims to provide students with more flexibility in their course selection while still incorporating English language instruction. By integrating English language learning within regular courses, students can develop their English skills in a more practical and contextual manner in applied mathematics and applications.
Mixing languages in a master track can offer several benefits:
- Language Proficiency
-
Students have the opportunity to improve their proficiency in both French and English, which are valuable skills in today’s globalized world. Developing competency in multiple languages can enhance students' communication abilities and open up international career prospects.
- Cultural Competence
-
Exposure to different languages and cultures enriches students' understanding and appreciation of diverse perspectives. It fosters a multicultural learning environment, promoting intercultural competence and broadening students' horizons.
- Career Opportunities
-
Proficiency in both French and English is often sought after by employers, particularly in international or multinational organizations. Having a mastery of both languages can provide graduates with a competitive edge in the job market.
- Multilingual Communication Skills
-
By requiring reports and oral presentations in English, students develop the ability to effectively communicate complex concepts in a second language. This skillset is highly valuable in professional settings where multilingual communication is required.
However, it’s important to ensure that
-
the language requirements and expectations are clearly communicated to students beforehand, so they can adequately prepare and manage their language learning.
-
we provide some support, such as language resources or language assistance programs, can also be beneficial to help students succeed in a multilingual environment.
Overall, a master track that combines French and English courses, along with opportunities for report writing and oral presentations in English, can offer a unique and attractive learning experience, preparing students for international collaboration and global career opportunities.
Furthermore, the Master CSMI program recognizes the potential to attract students from various European countries and beyond, taking advantage of its geographical proximity to Germany and Luxembourg. While the program previously had limited exposure to English-language courses, the curriculum changes in this document present an opportunity to appeal to a broader international student base.
By offering a combination of French and English courses, along with opportunities for report writing and oral presentations in English, the program can now cater to the needs of students from different linguistic backgrounds.
To ensure clear communication of language requirements and expectations to students:
-
Clearly outline language requirements in program documentation.
-
Include language-related information in course descriptions.
-
Organize orientation sessions to provide detailed information on language expectations.
-
Maintain ongoing communication with students about language requirements and updates.
-
Include explicit language expectations in course syllabi.
-
Ensure faculty are aware of language requirements and provide guidance.
-
Establish student support services for language assistance and practice through the use of modern technologies (not yet clear for example if we can use the language center of the university even though we don’t use the course facilities).
These measures help students prepare and manage their language learning effectively.
A short survey of the current Master track CSMI teachers (13 answers) shows that 46% are willing to teach in English, 38% may be willing to do it and only 10% are not willing to teach in English. This is a good sign for the future of the program since we would like to have both courses in french and english.
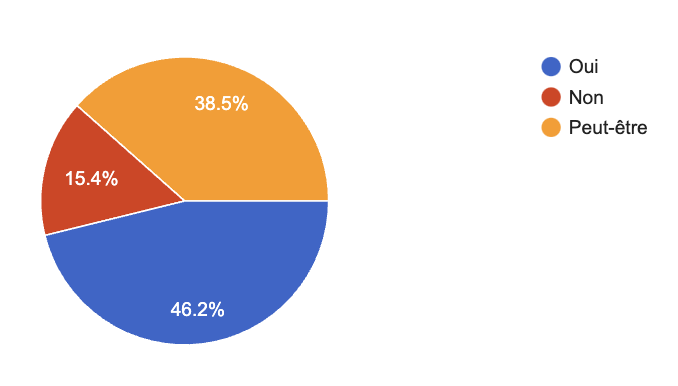
The next figure show that courses may be taught in English in various ways in about the same proportion: courses taught in english completely, course taught in french but the course notes are in english, exercices in english, oral presentations and written reports can be in english. We can leverage this to implement the plan above.
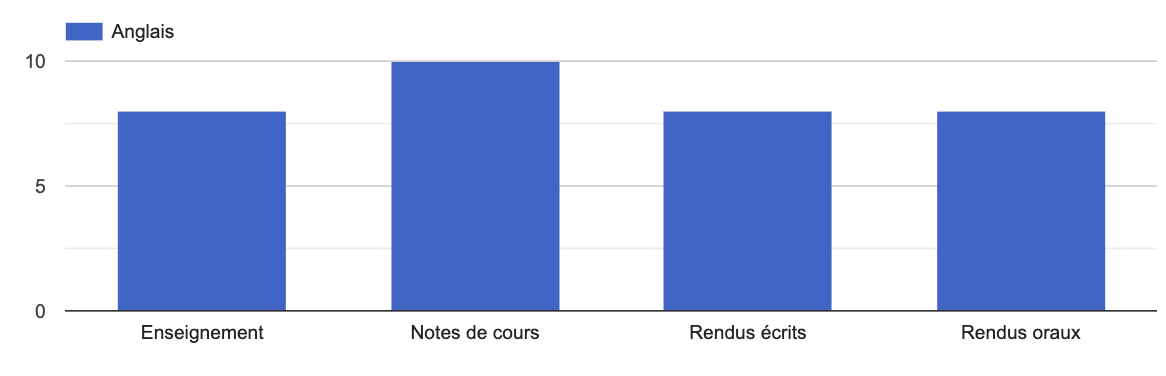
In the courses section we propose a list of courses that can be taught in english. We can see that the list is quite long and that we can easily have half of the courses per semester taught in english.
4.3. Introduction to Scientific Maching Learning
The SciML courses would ideally complement and bridge the knowledge provided by the standalone Machine Learning (ML) and Scientific Computing courses in a master’s track. Here’s a brief overview of how they might fit:
- ML Courses
-
These provide the fundamental concepts and techniques of machine learning. They would cover topics like linear regression, logistic regression, SVMs, neural networks, and more advanced topics like deep learning, reinforcement learning, and unsupervised learning methods. They would also discuss evaluation metrics, overfitting, underfitting, and other concepts relevant to ML model training and validation.
- Scientific Computing Courses
-
These would delve into the mathematical and computational methods used to model and solve scientific and engineering problems. Topics would include numerical methods, linear algebra, differential equations, optimization, and potentially high-performance computing.
- SciML Courses
-
These courses would serve as the bridge between the ML and Scientific Computing courses. They would take the machine learning techniques learned in the ML courses and apply them to the problems discussed in the Scientific Computing courses, and vice versa.
In terms of the order, it would be ideal for students to first complete the ML and Scientific Computing courses, as these will provide the foundational knowledge necessary for understanding and applying SciML. The SciML courses could then be taken towards the end of the master’s track, allowing students to apply all the knowledge they’ve gained in a novel and interdisciplinary way. The final projects in the SciML courses could potentially even serve as the basis for a master’s thesis or capstone project.
Scientific Machine Learning (SciML) courses can be highly beneficial for applied mathematicians in a master’s track for several reasons:
- Interdisciplinary Knowledge
-
SciML is inherently interdisciplinary, combining elements of computer science, applied mathematics, and domain-specific knowledge (e.g., physics, biology, etc.). This wide range of knowledge can be beneficial for applied mathematicians who want to apply their skills in various fields.
- Real-World Applications
-
SciML has a host of real-world applications. These range from climate modeling to drug discovery to predictive maintenance and more. This can provide applied mathematicians with a practical outlet for their skills.
- Cutting Edge
-
SciML is a relatively new and rapidly developing field. Being trained in this area can provide applied mathematicians with skills that are in high demand in both academia and industry.
- Data-Driven Modeling
-
Traditionally, applied mathematics has focused on model-driven approaches where mathematical models are derived based on understanding of the underlying phenomena. However, in many real-world problems, the phenomena are too complex to be fully captured by such models. In such cases, data-driven modeling, as used in SciML, can be a powerful tool.
- Improved Prediction and Generalization
-
Incorporating physical laws and principles into ML models, as is done in SciML, can lead to better generalization and predictive performance, particularly when data is scarce. This can be a valuable skill for applied mathematicians working on data-limited problems.
Here is an example as of May 2023 of a SciML course:
-
The course "Introduction to Scientific Machine Learning" at Purdue University provides an excellent framework for an introductory course in SciML. The course introduces data science to engineers with no prior knowledge and follows a probabilistic perspective that highlights the first principles behind the presented methods. It covers a variety of topics, including supervised learning, unsupervised learning, state space models, and physics-informed deep learning. It also offers training in various Python coding skills and commonly used data analytics software.
and here is an example of introductory course for ML:
-
Another comprehensive course that could serve as a reference is the edX course also from Purdue University, which provides an extensive review of probability theory, uncertainty propagation, supervised and unsupervised learning, state-space models, and automated Bayesian inference. It also covers advanced topics such as Gaussian process regression, neural networks, and advanced methods for characterizing posteriors. The prerequisites for this course include a working knowledge of multivariate calculus and basic linear algebra, basic Python knowledge, and familiarity with probability and numerical methods for engineering.
4.4. Blurring the line between Model driven versus data driven modeling and data assimilation
Model-driven modeling and data-driven modeling represent two different approaches to understanding and predicting system behavior. We plan on showing CSMI students how to use both approaches in their work, and how to combine them in a principled and practical way.
- Model-driven Modeling
-
This approach is based on the use of first principles, often derived from the physical sciences, to create a mathematical model of a system. The model incorporates known laws of physics, chemistry, or other relevant fields to predict system behavior. This method is powerful for situations where the underlying physical processes are well-understood and can be accurately represented mathematically. However, it can struggle in situations where the system is too complex or poorly understood to be accurately represented by a simplified mathematical model.
- Data-driven Modeling
-
This approach is based on the use of data and statistical methods to understand system behavior. In this case, a model is created based on observed data rather than on first principles. Machine learning techniques are often used to "learn" the model from the data. Data-driven modeling can be very effective in situations where there is a lot of high-quality data available, and it can capture complex, non-linear relationships that might be missed by a simpler model-driven approach. However, it can struggle in situations where data is scarce or noisy, and it can sometimes result in models that are difficult to interpret or that do not generalize well to new situations.
In the context of advanced courses at a master’s level, it can be beneficial to teach both model-driven and data-driven modeling techniques, as well as ways to combine the two approaches. This can be done in several ways:
- Model-informed Machine Learning
-
In this approach, physical models are used to inform the structure or training of machine learning models. For example, conservation laws or other physical principles can be used as constraints in the learning process. This can help to improve the accuracy and generalizability of machine learning models, particularly in situations where data is scarce or noisy.
- Machine Learning-enhanced Modeling
-
In this approach, machine learning models are used to enhance traditional model-driven modeling techniques. For example, machine learning models can be used to learn error terms or corrections to a physics-based model, or to model complex phenomena that are not well-captured by a simple physics-based model.
In these advanced courses, students can be exposed to the strengths and weaknesses of each approach, and learn how to choose and apply the most appropriate modeling techniques for a given problem. They can also learn how to integrate physical knowledge with machine learning in a principled way, which is a key aspect of Scientific Machine Learning. Practical exercises and projects that involve both model-driven and data-driven modeling can be a valuable part of these courses, providing students with hands-on experience of the techniques they are learning.
Data assimilation is another powerful method that blends model-driven and data-driven modeling, and it’s often used in fields such as meteorology, oceanography, and geophysics where both model predictions and observational data are available.
- Data Assimilation
-
This process involves combining observational data with the output of a predictive model to improve the model’s estimates of the state of a system. This is often done in a sequential manner, where the model’s state estimate is updated each time new data becomes available. The goal is to minimize the discrepancy between the model’s predictions and the actual observations, and to account for uncertainties in both the model and the data.
There are several methods of data assimilation, including:
- Kalman Filter
-
This is a recursive method used for estimating the state of a linear dynamic system from a series of measurements. It takes into account the uncertainties in the model and the measurements.
- Ensemble Kalman Filter
-
This is an extension of the Kalman filter which is used for non-linear systems. It uses a Monte Carlo approach to represent the probability distribution of the state estimate.
- 4D-Var (Four-Dimensional Variational data assimilation)
-
This method involves adjusting the model’s state at the start of a time window to minimize the discrepancy between the model’s predictions and the observations over the entire time window. This methodology stems for Control Theory.
In the context of master-level courses, data assimilation can be a valuable topic to cover, as it provides students with practical techniques for integrating model-driven and data-driven approaches. It also provides exposure to important concepts such as uncertainty quantification and state estimation. Coursework could include practical exercises involving the implementation and application of data assimilation methods, and could cover advanced topics such as the design of optimal observation systems.
4.5. High Performance Computing
High-Performance Computing (HPC) has been an essential tool for scientific research, industry, and technology development for several decades. The scale of computational power we can harness has grown rapidly, from gigaflops in the 1990s to petaflops in the 2010s, and we are now on the brink of the exascale era.
Programs like NumPEx, the French initiative for Exascale computing, reflect this trend and underscore the importance of preparing for the next wave of computational capabilities [1]. NumPEx aims to design and develop the methods, algorithms and software components for future exascale machines and prepare major scientific and industrial application domains to fully exploit the capabilities of these machines.
This evolution in computing power necessitates an evolution in the skills and tools we use to harness it. The architectures of modern supercomputers are becoming more complex, with an increasing variety of hardware components that need to be programmed and optimized. The programming models we use to write parallel and distributed applications are also evolving, requiring programmers to understand and mix different programming paradigms, such as MPI, multi-threading, and GPU programming. Runtime execution systems are becoming crucial for managing the execution of programs on complex hardware.
To prepare for this future, we offer a sequence of three courses on High-Performance Computing as part of the CSMI Master’s program. These courses will give students a deep understanding of modern HPC, from the basics of parallel programming and numerical methods to the advanced techniques of hybrid computing and runtime systems. The courses will also introduce students to state-of-the-art HPC tools and frameworks, such as PETSc, that will be essential for tackling the computational challenges of the future.
These courses are not just about learning the theory of HPC; they’re about gaining practical skills that will equip students to contribute to the next wave of breakthroughs in scientific research, technology development, and industry, powered by exascale computing.
Course | Description |
---|---|
HPC 1: Foundations of High-Performance Computing |
This foundational course introduces students to the architectures of modern supercomputers, focusing on CPUs and the interconnect, and the basics of parallel programming with the Message Passing Interface (MPI). We will also introduce numerical methods for scientific computation and the Portable, Extensible Toolkit for Scientific Computation (PETSc [2]) as a general framework for implementing these methods in an HPC context. |
HPC 2: Advanced Techniques in High-Performance Computing |
Building on HPC 1, this course delves deeper into parallel computing, focusing on multi-threading techniques using OpenMP and GPU programming with CUDA or OpenCL. Students will learn how to leverage the power of multi-core processors and GPUs to accelerate their computations. We will continue our exploration of PETSc on top of Nvidia Cuda and AMD Hip, showing how it can be used to manage complex multi-threaded and GPU-accelerated computations. |
HPC 3: High-Performance Computing for the Future |
The final course will cover the concept of hybrid computing, which involves mixing different programming models and hardware in a single program, and runtime execution systems that manage the execution of programs on complex, heterogeneous hardware. Performance analysis, optimization, parallel I/O and data management will also be covered. We will discuss how frameworks like PETSc can be used in this context to help manage the complexity of hybrid computing and runtime systems. |
4.6. Courses
Here’s a proposed modification to the semester courses of CSMI:
Course | ECTS | Type | Topics | Semester | Teacher | Language |
---|---|---|---|---|---|---|
Semester 1 |
||||||
Operating System |
3 (28h equiv. TD) |
CI |
BASE |
S1 |
P. David |
Fr |
Algorithmics & Graphs |
3 (28h equiv. TD) |
CI |
BASE |
S1 |
Y Bugeaud |
En, Fr |
Project 1 |
3 (28h equiv. TD) |
CI |
BASE |
S1 |
C. Prud’homme, J. Aghili |
En, Fr |
Data Processing and Mining |
3 (28h equiv. TD) |
CI |
BASE |
S1 |
V. Vigon |
En, Fr |
Database |
3 (28h equiv. TD) |
CI |
HPC |
S1 |
Ext. Guest Lecturer |
En, Fr |
C++ |
3 (28h equiv. TD) |
CI |
HPC |
S1 |
V. Chabannes |
Fr |
High-Performance Computing 1 |
3 (28h equiv. TD) |
CI |
HPC |
S1 |
Ext. B. Bramas, V. Chabannes, M. Boileau |
En, Fr |
Scientific Computing 1 |
3 (28h equiv. TD) |
CI |
BASE |
S1 |
P. Helluy |
En, Fr |
Scientific Computing 2 |
3 (28h equiv. TD) |
CI |
MSO |
S1 |
L. Navoret |
En, Fr |
Random Models |
3 (28h equiv. TD) |
CI |
MSO |
S1 |
V. Vigon |
Fr |
Semester 2 |
||||||
Signal and Image Processing 1 |
3 (28h equiv. TD) |
CI |
DATA |
S2 |
V. Vigon |
Fr |
Scientific Machine Learning 1 |
3 (28h equiv. TD) |
CI |
ML / ROM |
S2 |
L. Navoret |
En, Fr |
Numerical Methods for PDE 1 |
6 (56h equiv. TD) |
CI |
MSO |
S2 |
C. Prud’homme, J. Aghili |
En, Fr |
Optimization |
6 (56h equiv. TD) |
CI |
MSO |
S2 |
L. Navoret |
En, Fr |
High-Performance Computing 2 |
3 (28h equiv. TD) |
CI |
HPC |
S2 |
Ext. B. Bramas, V. Chabannes, M. Boileau |
En, Fr |
Project 2 |
3 (28h equiv. TD) |
CI |
BASE |
S2 |
C. Prud’homme |
En, Fr |
Internship or Thesis |
6 (56h equiv. TD) |
Internship |
BASE |
S2 |
Program Coordinator |
En, Fr |
Semester 3 |
||||||
ROM & Data-Driven ROM |
3 (28h equiv. TD) |
CI |
ML / ROM |
S3 |
C. Prud’homme |
En, Fr |
Optimal Control |
6 (56h equiv. TD) |
CI |
MSO |
S3 |
B. Rao, Ext. M. Duprez, C. Prud’homme |
En, Fr |
Numerical Methods for PDE 2 |
3 (28h equiv. TD) |
CI |
MSO |
S3 |
P. Helluy |
En, Fr |
High-Performance Computing 3 |
3 (28h equiv. TD) |
CI |
HPC |
S3 |
Ext. B. Bramas, V. Chabannes, M. Boileau |
En, Fr |
Uncertainties |
3 (28h equiv. TD) |
CI |
MSO |
S3 |
E. Birmelé |
En, Fr |
Signal and Image Processing 2 |
3 (28h equiv. TD) |
CI |
DATA |
S3 |
V. Vigon |
Fr |
Scientific Machine Learning 2 |
3 (28h equiv. TD) |
CI |
ML / ROM |
S3 |
E. Franck |
En, Fr |
Network-Integrated Pre and Post-Processing in Scientific Computing |
3 (28h equiv. TD) |
CI |
MSO |
S3 |
Ext. C. Trophime, J. Sarton |
En, Fr |
Project 3 |
3 (28h equiv. TD) |
CI |
BASE |
S3 |
C. Prud’homme, J. Aghili |
En, Fr |
Semester 4 |
||||||
Internship or Thesis |
30 |
Internship |
BASE |
S4 |
Program Coordinator |
En, Fr |
In this modification, we added three courses on high-performance computing, which covers various topics such as multigrid, domain decomposition, MPI, OpenMP, CUDA, and optimization of codes for high-performance computing architectures. We also added two courses on machine learning, one on scientific machine learning. Finally, we included a course on data assimilation and digital twins in the third semester.
4.6.1. Semester 1
- Operating system
-
This course delves into the principles of operating systems, focusing on process and memory management, file systems, and I/O handling. For Applied Mathematics students, this knowledge is essential as it allows for the efficient execution of mathematical computations and simulations on computer systems, and provides a foundation for understanding how software interacts with hardware.
- Algorithmics and Graphs
-
This course provides a deep understanding of algorithm design and analysis, including data structures, sorting algorithms, graph algorithms, and dynamic programming. It equips Applied Mathematics students with the skills to implement mathematical algorithms efficiently, which is crucial for problem-solving in various mathematical fields.
- Project 1
-
This course introduces students to project management and programming environments. It covers modern software project management techniques, Continuous Integration, Delivery, Deployment, container technologies, and the usage of cloud environments like GitHub, Azure, or AWS. The course includes a practical project, providing Applied Mathematics students with hands-on experience in managing and implementing software projects. This course provides the basis for the projects and internships in the following semesters.
- Data Processing and Mining
-
This course provides a comprehensive understanding of data processing and mining, including data cleaning, pre-processing, feature selection, clustering, and classification. Applied Mathematics students will gain the skills to analyze large datasets efficiently, which is crucial in fields such as data science, machine learning, and statistical analysis.
- Database
-
This course delves into the principles of database design and implementation, including relational database models, SQL, and NoSQL. For Applied Mathematics students, this knowledge is essential for the efficient storage and retrieval of data, which is a key component of many fields including data analysis, machine learning, and software development.
- C++
-
This course provides a comprehensive understanding of C++ programming, including object-oriented programming, templates, and the Standard Template Library. Applied Mathematics students will gain the skills to implement mathematical algorithms efficiently, which is crucial for problem-solving in various mathematical fields.
- High-Performance Computing 1
-
This foundational course introduces students to the architectures of modern supercomputers, focusing on CPUs and the interconnect, and the basics of parallel programming with the Message Passing Interface (MPI). We will also introduce numerical methods for scientific computation and the Portable, Extensible Toolkit for Scientific Computation (PETSc) as a general framework for implementing these methods in an HPC context. Students will gain the skills to implement mathematical algorithms on high-performance computing systems, which is crucial for fields such as computational mathematics, data science, and machine learning.
- Scientific Computing 1
-
This course provides a comprehensive understanding of scientific computing, including the finite difference method. Applied Mathematics students will gain the skills to implement mathematical algorithms on computer systems, which is crucial for fields such as computational mathematics, data science, and machine learning.
- Scientific Computing 2
-
This course provides a comprehensive understanding of advanced scientific computing topics, including sparse linear system solvers, resolution of sparse eigenvalue systems, numerical methods for partial differential equations in 1D using finite elements, and advanced Python programming. Applied Mathematics students will gain the skills to implement mathematical algorithms on computer systems, which is crucial for fields such as computational mathematics, data science, and machine learning.
- Random Models
-
This course provides a deep understanding of random models, including the Law of Large Numbers, Central Limit Theorem, Monte Carlo method, rejection method, scale invariance, isotropy, stochastic homogeneity, random walk, Brownian motion, diffusion, stochastic differential calculus, Markov chains, MCMC, particle systems, and simulated annealing. Applied Mathematics students will gain the skills to model stochastic processes efficiently, which is crucial for fields such as financial mathematics, industrial mathematics, risk analysis, and machine learning.
4.6.2. Semester 2
- Signal and Image Processing 1
-
This course provides a comprehensive understanding of signal and image processing, including 1D (sound) and 2D (image) signals, sampling, Fourier series, FFT filtering, time-frequency analysis, aliasing phenomena, chromatic spaces, quantization, edge detection, denoising, segmentation, JPEG compression, and image calibration. Applied Mathematics students will gain the skills to analyze signals and images efficiently, which is crucial for fields such as data science, machine learning, and multimedia processing.
- Scientific Machine Learning 1
-
This course provides a deep understanding of scientific machine learning, including supervised and unsupervised learning, deep learning, and generative models. Applied Mathematics students will gain the skills to analyze large datasets efficiently, which is crucial for fields such as data science, machine learning, and statistical analysis.
- Numerical Methods for PDE
-
This course provides a comprehensive understanding of numerical methods for partial differential equations, particularly the finite element method and the study of various standard PDEs from a theoretical and pratical point of view. Applied Mathematics students will gain the skills to implement mathematical algorithms on computer systems, which is crucial for fields such as computational mathematics and sciences.
- Optimization
-
This course provides a deep understanding of optimization, including convex analysis, solution existence and uniqueness theorems, optimality conditions such as Euler equations and inequalities, Lagrange multipliers, Kuhn-Tucker relations, saddle point and duality theory, and numerical algorithms including gradient, relaxation, Newton and quasi-Newton, penalization, and Uzawa methods. Applied Mathematics students will gain the skills to implement mathematical algorithms efficiently, which is crucial for fields such as operations research, machine learning, and industrial mathematics.
- High-Performance Computing 2
-
Building on HPC 1, this course delves deeper into parallel computing, focusing on multi-threading techniques using OpenMP and GPU programming with CUDA, HIP or OpenCL. Students will learn how to leverage the power of multi-core processors and GPUs to accelerate their computations. We will continue our exploration of PETSc, showing how it can be used to manage complex multi-threaded and GPU-accelerated computations. Applied Mathematics students will gain the skills to implement mathematical algorithms on high-performance computing systems, which is crucial for fields such as computational mathematics, data science, and machine learning.
- Project 2
-
This course involves collaborative projects with academia or enterprises, providing students with hands-on experience in applying their theoretical knowledge and skills in research-oriented and real-world industry settings. This experience is invaluable for Applied Mathematics students as it provides a practical context for their theoretical knowledge.
- Internship
-
A 2-month internship in a company or research laboratory provides Applied Mathematics students with practical experience in a professional setting, allowing them to apply their theoretical knowledge and skills in real-world scenarios.
4.6.3. Semester 3
- ROM & Data driven ROM
-
This course provides a deep understanding of reduced order modeling and data driven reduced order modeling, including proper orthogonal decomposition, reduced basis methods, data assimilation and data driven ROM. Applied Mathematics students will gain the skills to implement mathematical algorithms efficiently, which is crucial for fields such as computational mathematics, machine learning, control systems and various computational sciences.
- Optimal Control
-
This course provides a comprehensive understanding of optimal control, including Pontryagin’s maximum principle, dynamic programming, data assimilation, and numerical methods for optimal control. Applied Mathematics students will gain the skills to implement mathematical algorithms efficiently, which is crucial for fields such as control systems, operations research, and industrial mathematics.
- Numerical Methods for PDE 2
-
This course provides a deep understanding of numerical methods for partial differential equations, particularly the finite volume method and DG methods for hyperbolic systems. Applied Mathematics students will gain the skills to implement mathematical algorithms on computer systems, which is crucial for fields such as computational mathematics, fluid dynamics, and mathematical physics.
- High-Performance Computing 3
-
The final course will cover the concept of hybrid computing, which involves mixing different programming models and hardware in a single program, and runtime execution systems that manage the execution of programs on complex, heterogeneous hardware. Performance analysis, optimization, parallel I/O and data management will also be covered. We will discuss how PETSc can be used in this context to help manage the complexity of hybrid computing and runtime systems. Applied Mathematics students will gain the skills to implement mathematical algorithms on high-performance computing systems, which is crucial for fields such as computational mathematics, data science, and machine learning.
- Uncertainties
-
This course provides a deep understanding of uncertainties, including stochastic modeling, uncertainty quantification, and sensitivity analysis. Applied Mathematics students will gain the skills to model stochastic processes efficiently, which is crucial for fields such as industrial mathematics, risk analysis, and machine learning.
- Signal and Image Processing 2
-
This course provides a comprehensive understanding of advanced signal and image processing, including classification, segmentation, and generation of images, sounds, and text using deep learning techniques. Applied Mathematics students will gain the skills to analyze signals and images efficiently, which is crucial for fields such as data science, machine learning, and multimedia processing.
- Scientific Machine Learning 2
-
This course provides a deep understanding of scientific machine learning, including supervised and unsupervised learning, deep learning, and generative models. Applied Mathematics students will gain the skills to analyze large datasets efficiently, which is crucial for fields such as data science, machine learning, and statistical analysis.
- Network-Integrated Pre and PostProcessing in Scientific Computing
-
This course provides a comprehensive understanding of pre and post processing in scientific computing, including geometry and mesh generation, mesh adaptation, visualization, and data analysis. Applied Mathematics students will gain the skills to implement mathematical algorithms on computer systems, which is crucial for fields such as computational mathematics, data science,and machine learning. There will be an emphasis on the integration of these processes with network data access. Students gain practical knowledge and interdisciplinary skills to effectively manage network-based data acquisition, processing, and storage, while developing problem-solving, programming, data analysis, and collaboration skills for efficient and professional application in real-world scenarios. More details here.
- Project 3
-
This course involves collaborative projects with academia or enterprises, providing students with hands-on experience in applying their theoretical knowledge and skills in research-oriented and real-world industry settings. This experience is invaluable for Applied Mathematics students as it provides a practical context for their theoretical knowledge.
4.6.4. Semester 4
- Internship
-
A 6-month internship in a company or research laboratory provides Applied Mathematics students with practical experience in a professional setting, allowing them to apply their theoretical knowledge and skills in real-world scenarios. This experience is invaluable for Applied Mathematics students as it provides a practical context for their theoretical knowledge and prepares them for their future careers.
4.7. Skills set to be acquired by CSMI Students
- Problem-Solving Skills
-
The ability to apply mathematical and computational methods to solve complex problems.
- Programming Skills
-
Proficiency in programming languages --- C/C++, Python and Rust --- and understanding of software development principles.
- Data Analysis Skills
-
The ability to process, analyze, and interpret large datasets using various data analysis techniques.
- Algorithm Design and Analysis
-
Understanding of how to design, implement, and analyze algorithms for various computational problems.
- High-Performance Computing
-
Understanding of how to optimize and parallelize computations to run efficiently on high-performance computing systems.
- Database Management
-
Understanding of how to design, implement, and manage databases.
- Machine Learning
-
Understanding of various machine learning techniques and their applications.
- Signal and Image Processing
-
Understanding of how to analyze and process signals and images.
- Project Management
-
Understanding of how to manage software projects, including the use of modern software project management techniques and tools.
- Communication Skills
-
The ability to effectively communicate complex mathematical and computational concepts, both orally and in writing.
- Collaboration Skills
-
The ability to work effectively in a team, including in collaborative projects with academia and enterprises.
- Research Skills
-
The ability to conduct independent research, including the ability to read and understand academic papers, and to design and implement research projects.
- Professional Skills
-
Understanding of professional practices, including ethical considerations, and the ability to apply theoretical knowledge in practical, real-world settings through internships.
4.8. Courses and Skills
The following is present the courses with the skills acquired by the students in the CSMI program:
Courses | Skills Acquired |
---|---|
Semester 1 |
|
Operating system |
Problem-Solving Skills, High-Performance Computing, Database Management |
Algorithmics and Graphs |
Problem-Solving Skills, Algorithm Design and Analysis |
Project 1 |
Project Management, Programming Skills, Collaboration Skills, Problem-Solving Skills |
Data Processing and Mining |
Data Analysis Skills, Machine Learning |
Database |
Database Management |
C++ |
Programming Skills, Problem-Solving Skills |
High-Performance Computing 1 |
High-Performance Computing, Problem-Solving Skills |
Scientific Computing 1 |
High-Performance Computing, Problem-Solving Skills |
Scientific Computing 2 |
High-Performance Computing, Problem-Solving Skills |
Random Models |
Problem-Solving Skills, Machine Learning |
Semester 2 |
|
Signal and Image Processing 1 |
Signal and Image Processing, Data Analysis Skills, Machine Learning |
Scientific Machine Learning 1 |
Data Analysis Skills, Machine Learning |
Numerical Methods for PDE |
High-Performance Computing, Problem-Solving Skills |
Optimization |
Problem-Solving Skills, Algorithm Design and Analysis |
High-Performance Computing 2 |
High-Performance Computing, Problem-Solving Skills |
Project 2 |
Potentially all skills but in particular Project Management, Collaboration Skills, Communication Skills, Problem-Solving Skills, Professional Skills |
Internship |
Potentially all skills but in particular Project Management, Professional Skills, Collaboration Skills, Problem-Solving Skills, Communication Skills |
Semester 3 |
|
ROM & Data driven ROM |
High-Performance Computing, Problem-Solving Skills |
Optimal Control |
Problem-Solving Skills, Optimization |
Numerical Methods for PDE 2 |
High-Performance Computing, Problem-Solving Skills |
High-Performance Computing 3 |
High-Performance Computing, Problem-Solving Skills |
Uncertainties |
Problem-Solving Skills, Machine Learning |
Signal and Image Processing 2 |
Signal and Image Processing, Data Analysis Skills, Machine Learning |
Scientific Machine Learning 2 |
Data Analysis Skills, Machine Learning |
Pre and PostProcessing in Scientific Computing |
High-Performance Computing, Problem-Solving Skills |
Networks |
High-Performance Computing, Problem-Solving Skills |
Project 3 |
Potentially all skills but in particular Project Management, Collaboration Skills, Communication Skills, Problem-Solving Skills, Professional Skills |
Semester 4 |
|
Internship |
Potentially all skills but in particular Project Management, Professional Skills, Collaboration Skills, Problem-Solving Skills, Communication Skills |
We now break down the macro skills into micro skills in the following tables
4.8.1. Micro skills breakdown
Course | Macro Skills | Micro Skills |
---|---|---|
Operating System |
Problem-Solving Skills, High-Performance Computing, Database Management |
|
Algorithmics and Graphs |
Problem-Solving Skills, Algorithm Design and Analysis |
|
Project 1 |
Project Management, Programming Skills, Collaboration Skills |
|
Data Processing and Mining |
Data Analysis Skills, Machine Learning |
|
Database |
Database Management |
|
C++ |
Programming Skills, Problem-Solving Skills |
|
High-Performance Computing 1 |
High-Performance Computing, Problem-Solving Skills |
|
Scientific Computing 1 |
High-Performance Computing, Problem-Solving Skills |
|
Scientific Computing 2 |
High-Performance Computing, Problem-Solving Skills |
|
Random Models |
Problem-Solving Skills, Machine Learning |
|
Course | Macro Skills | Micro Skills |
---|---|---|
Signal and Image Processing 1 |
Signal and Image Processing, Data Analysis Skills, Machine Learning |
|
Scientific Machine Learning 1 |
Data Analysis Skills, Machine Learning |
|
Numerical Methods for PDE |
High-Performance Computing, Problem-Solving Skills |
|
Optimization |
Problem-Solving Skills, Algorithm Design and Analysis |
|
High-Performance Computing 2 |
High-Performance Computing, Problem-Solving Skills |
|
Project 2 |
Project Management, Collaboration Skills |
|
Internship |
Project Management, Professional Skills, Collaboration Skills, Problem-Solving Skills |
|
Course | Macro Skills | Micro Skills |
---|---|---|
ROM & Data driven ROM |
High-Performance Computing, Problem-Solving Skills |
|
Optimal Control |
Problem-Solving Skills, Optimization |
|
Numerical Methods for PDE 2 |
High-Performance Computing, Problem-Solving Skills a |
- Implement the finite volume method - Apply discontinuous Galerkin methods for hyperbolic systems - Use numerical methods to solve PDEs |
High-Performance Computing 3 |
High-Performance Computing, Problem-Solving Skills |
|
Uncertainties |
Problem-Solving Skills, Machine Learning |
|
Signal and Image Processing 2 |
Signal and Image Processing, Data Analysis Skills, Machine Learning |
|
Scientific Machine Learning 2 |
Data Analysis Skills, Machine Learning |
|
Pre and PostProcessing in Scientific Computing |
High-Performance Computing, Problem-Solving Skills |
|
Networks |
High-Performance Computing, Problem-Solving Skills |
|
Project 3 |
Project Management, Collaboration Skills |
|
Course | Macro Skills | Micro Skills |
---|---|---|
Internship |
Project Management, Professional Skills, Collaboration Skills, Problem-Solving Skills |
|
5. ChangeLog
5.1. v1.4 - 2023-06-19
-
adopted the course "Network-Integrated Pre and Post Processing in Scientific Computing" instead of the two courses on "Networks" and "Pre and Post-Processing in Scientific Computing
-
Y. Bugeaud will teach the course "Algorithmics and Graphs"
-
Indicated colleagues external to the UFR by prefixing with Ext. their names.
5.2. v1.3 - 2023-06-07
-
Added a more substantial discussion in section English Courses.
-
Updated the courses list by adding a language column to indicate the possible languages (english or french) for each course.
-
Added a section on Discussion on Networks and Pre and Post-Processing in Scientific Computing Courses to discuss a proposition to have a course on Network-Integrated Pre and Post Processing in Scientific Computing instead of the two courses on Networks and Pre and Post-Processing in Scientific Computing.
-
Updated the list of macro skills for projects and internships.
5.3. v1.2 - 2023-06-06
-
Added a Changelog section
-
Added the section on High Performance Computing and updated the HPC course descriptions [HPC1] [HPC2] and [HPC3] accordingly.
5.4. v1.1 - 2023-06-05
-
Updated section on Courses and Skills to include the micro skills breakdown for each course. This section will need to be updated as the course descriptions are finalized.
Appendix A: Discussion on Networks and Pre and Post-Processing in Scientific Computing Courses
The following is a discussion on the Network and Pre and Post-Processing in Scientific Computing courses, which are proposed as alternatives for the third semester of the CSMI program. Instead of taking either courses, we can provide the course "Network-Integrated Pre and Post Processing in Scientific Computing".
A.1. Course offer
This course offers a detailed exploration of pre and post-processing techniques in scientific computation, with a particular focus on integrating these processes with network data access. It covers key topics including geometry and mesh creation, mesh modification, data visualization and analysis, and efficient use of network frameworks and APIs for streamlined data transmission.
The course underscores the practicality of incorporating network connectivity to source data from varied inputs such as sensors, images, and simulation results. It provides students with hands-on experience in managing network-based data acquisition, processing, and storage, enabling them to apply mathematical algorithms and execute data-driven pre and post-processing operations.
Designed for students in computational mathematics, data science, machine learning, and related disciplines, this course sets the stage for an introduction to High Performance Data Analysis (HPDA). It aims to empower students with the ability to leverage network functionalities for a seamless integration of data into their computational workflows.
Key benefits of the course include:
- Practical Knowledge
-
Gain hands-on experience with network-based data acquisition, processing, and storage.
- Interdisciplinary Skills
-
Learn techniques relevant to computational mathematics, data science, machine learning, and more.
- Real-World Relevance
-
Understand how to apply these skills in real-world scenarios, particularly in the context of HPDA.
- Future-Proof Learning
-
Acquire skills that are in-demand and applicable to emerging technologies such as the Internet of Things (IoT).
- Enhanced Problem Solving
-
Develop a comprehensive understanding of pre and post-processing in scientific computing.
- Efficiency
-
Learn how to use network frameworks and APIs for efficient data transmission.
A.2. Integration in CSMI
The "Network-Integrated Pre and Post Processing in Scientific Computing" course, as we discussed earlier, is designed to provide a comprehensive understanding of pre and post-processing in scientific computing, while integrating data access through networks. Looking at the course list, there are several ways this course integrates into the master track:
- Building on Foundational Courses
-
In Semester 1, students learn the basics of operating systems, algorithmics, data processing and mining, and databases. The proposed course builds on these foundational skills, teaching students how to apply these concepts in a networked environment for scientific computing.
- Complementing Advanced Courses
-
Courses like "High-Performance Computing" and "Scientific Computing" in Semester 1 and 2, focus on advanced computing techniques and algorithms. The proposed course complements these courses by teaching students how to efficiently handle and process data, a critical aspect of high-performance and scientific computing.
- Integration with Data-Centric Courses
-
The course nicely ties in with data-centric courses like "Data Processing and Mining", "Scientific Machine Learning", and "Signal and Image Processing". The skills learned in these courses can be used in conjunction with the network integration skills taught in the proposed course to manage, analyze, and visualize data more effectively.
- Preparation for Practical Applications
-
The course provides practical, hands-on experience, which will be useful for students during their projects and internship or thesis work in Semesters 2, 3, and 4.
- Introduction to HPDA
-
This course introduces students to the concept of High Performance Data Analysis (HPDA), which could be a valuable supplement to the other high-performance computing and machine learning courses in the curriculum.
Overall, the proposed course can be a strong addition to the master track, providing a valuable set of skills that complements and enhances the other courses in the curriculum.
A.3. Course skills
The following skills would apply to the "Network-Integrated Pre and Post Processing in Scientific Computing" course:
- Problem-Solving Skills
-
The course includes a focus on implementing mathematical algorithms and performing data-driven pre and post-processing operations.
- Programming Skills
-
While the specific languages aren’t currently mentioned in the course description, it’s likely that some level of programming proficiency would be necessary for the practical aspects of the course.
- Data Analysis Skills
-
The course involves working with data from various sources, including sensors and images, and performing data analysis.
- High-Performance Computing
-
The course introduces students to High Performance Data Analysis (HPDA), which involves optimizing computations to run efficiently on high-performance computing systems.
- Communication Skills
-
As with most academic courses, the ability to effectively communicate complex concepts, both orally and in writing, would be beneficial.
- Collaboration Skills
-
The course involves hands-on experience, which could involve group projects requiring teamwork and collaboration.
- Research Skills
-
While not explicitly stated in the course description, research skills could be beneficial in understanding and implementing the latest methods in pre and post-processing, network integration, and HPDA.
- Professional Skills
-
The course has a practical emphasis, and the skills learned could be directly applicable in real-world settings, particularly those involving network-based data acquisition and processing.
It’s important to note that the specific skills required or developed might depend on the specific course content and structure, which could vary based on the instructor’s approach and the specific projects or assignments included in the course. This course will be taught by several colleagues as it involves various expertises. |
Here are some additional skills that could be directly associated to the course:
-
Implement mathematical algorithms for pre and post-processing tasks.
-
Analyze data collected from various networked sources to inform decision-making.
-
Optimize computations for efficient execution on high-performance computing systems.
-
Collaborate in team-based projects involving data acquisition, processing, and storage.
-
Apply professional practices in network-based data acquisition and processing in real-world settings.